Rynus Requesters are individuals or entities seeking scalable, cost-effective, and powerful computing resources for AI Training or Data Labeling tasks.
AI Training Requester
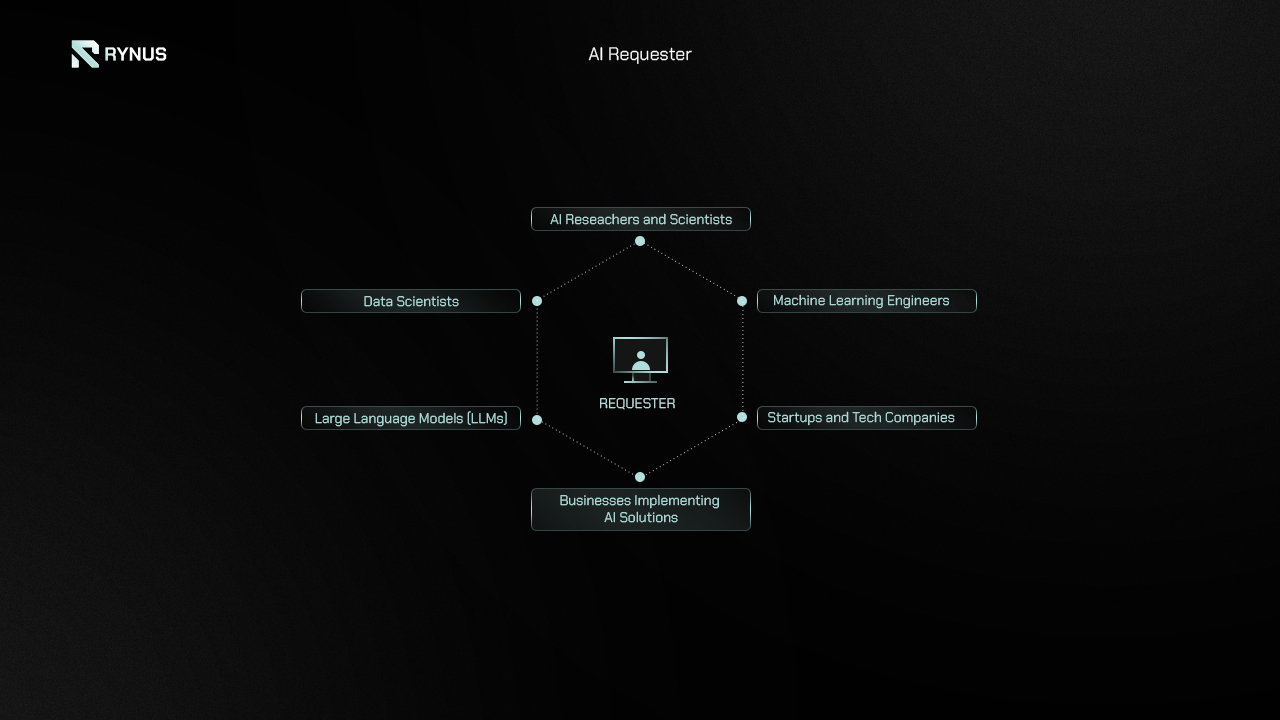
In the field of AI, the Requesters using Rynus’s services encompass a broad spectrum of individuals and organizations that require substantial cloud computing power to train their AI models. These include:
- AI Researchers and Scientists: These professionals work in academic institutions, research labs, and tech companies, focusing on advancing the state-of-the-art in artificial intelligence. They require extensive computational resources to experiment with novel algorithms, perform large-scale simulations, and validate their hypotheses through rigorous testing.
- Machine Learning Engineers: Employed in industries ranging from finance to healthcare, these engineers develop and deploy machine learning models for various applications. They need scalable and powerful computing infrastructure to train models efficiently, handle large datasets, and iterate quickly on their designs to optimize performance.
- Data Scientists: Working in diverse sectors like retail, telecommunications, and marketing, data scientists use cloud computing resources to analyze vast amounts of data, extract insights, and build predictive models. Rynus provides them with the necessary computational power to perform complex data processing and machine learning tasks.
- Startups and Tech Companies: Many startups and tech companies, particularly those focusing on AI-driven products and services, rely on cloud computing to scale their operations without significant upfront investment in hardware. These companies leverage Rynus’s decentralized network to access cost-effective and high-performance computing resources.
- Developers of Large Language Models (LLMs): Teams working on LLMs such as GPT, BERT, and others require immense computational power to train and fine-tune these models. Rynus offers a scalable solution to meet their high demands for GPU resources, enabling the development of sophisticated natural language processing tools.
- Businesses Implementing AI Solutions: Companies across various industries are integrating AI to enhance their services and products. From recommendation systems in e-commerce to fraud detection in banking, these businesses utilize Rynus to gain access to the necessary computing power for deploying and maintaining their AI models.
Diverse Pipelines, Outsourcing, and Remote Working
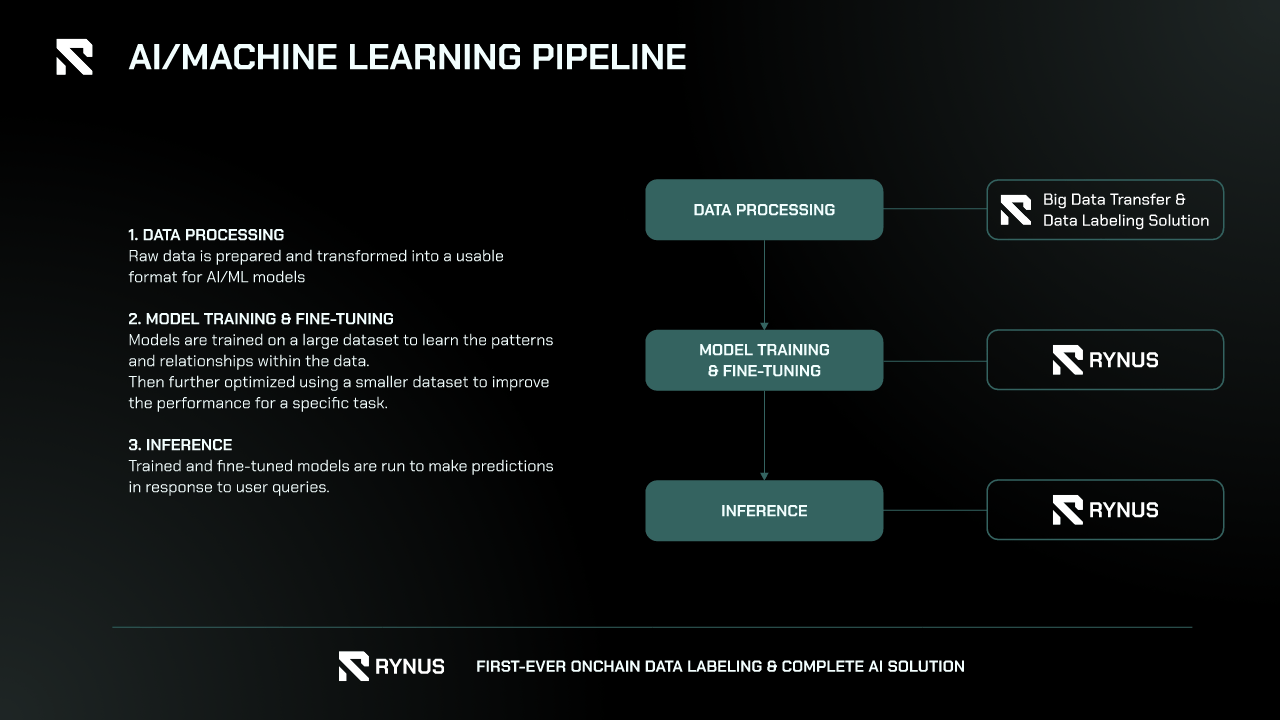
The AI field is characterized by diverse and complex pipelines that encompass various stages, from data collection and preprocessing to model training, validation, and deployment. AI projects often require collaboration among geographically dispersed teams, which can introduce significant challenges in terms of resource management and operational efficiency. Outsourcing and remote working have become prevalent, as organizations seek to leverage specialized skills from around the world, but these practices also bring about challenges related to infrastructure accessibility and resource allocation.
Rynus addresses these challenges effectively through three key strategies:
- Support for All AI SDKs and Libraries: Rynus provides extensive support for a wide range of AI software development kits (SDKs) and libraries, ensuring compatibility with popular tools and frameworks used by AI professionals. This includes support for TensorFlow, PyTorch, Keras, and other major libraries, allowing developers to seamlessly integrate their existing workflows into the Rynus ecosystem. By accommodating diverse toolsets, Rynus simplifies the process of transitioning to their platform and enhances productivity for AI teams working with various technologies.
- Provision of Unlimited Powerful GPUs: One of the core strengths of Rynus is its ability to offer unlimited access to powerful GPUs. This scalability ensures that AI projects, regardless of their size and complexity, can be executed without constraints on computational resources. Whether it’s training large-scale deep learning models or performing intensive data analysis, Rynus’s infrastructure can handle the demands, providing the necessary computational power to meet project deadlines and performance benchmarks.
- Seamless Global Accessibility: Rynus ensures seamless global accessibility, enabling AI professionals to access high-performance computing resources from anywhere in the world. This global reach is crucial for teams that operate remotely or collaborate across different time zones. By eliminating geographical barriers, Rynus facilitates real-time collaboration and resource sharing, making it easier for remote teams to work together efficiently on AI projects.
Flexible Pricing Models to Serve Both Enterprises and Individuals
To cater to a broad spectrum of users, from large enterprises to individual developers, Rynus offers two flexible pricing models:
- Pay-As-You-Go: This model allows users to pay only for the computing power they actually use. It’s an ideal option for individuals or organizations with variable workloads, as it provides cost-efficiency by aligning expenses with actual usage. Users can scale their computing resources up or down based on project requirements without being locked into long-term commitments.
- Rental Plan: For users with more consistent and predictable workloads, Rynus offers rental plans. These plans provide access to computing resources at a fixed cost, making it easier to budget for long-term projects. This model is particularly beneficial for enterprises that need to maintain continuous operations and require a steady supply of computational power.
In summary, Rynus meets the diverse needs of these Requesters, enabling them to harness AI’s full potential while optimizing costs and efficiency.
Data Labeling Requester
Data Labeling Requesters are typically organizations, research institutions, and AI-driven businesses that need accurately labeled data to train, test, and improve machine learning models. As AI and machine learning continue to expand into various fields, the demand for high-quality labeled data grows.
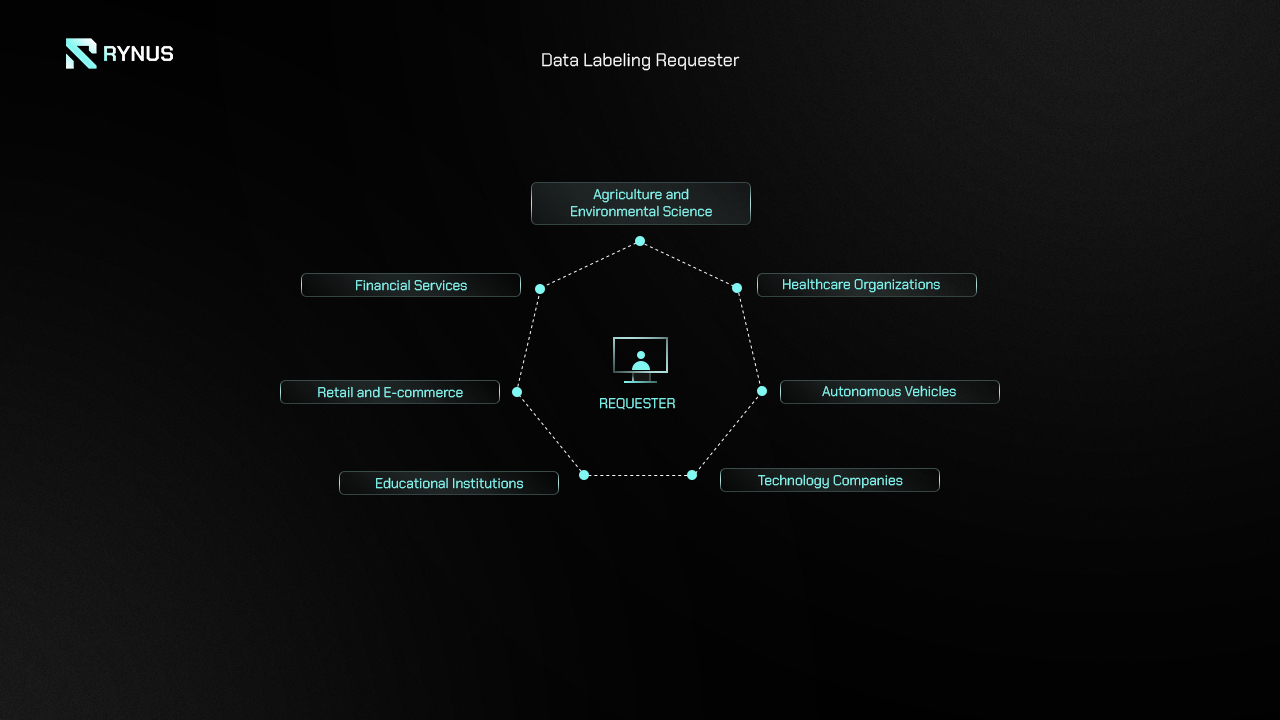
Data Labeling Requesters come from diverse industries, including:
- Technology Companies: Businesses that develop AI products, such as image recognition, natural language processing, and predictive analytics, rely heavily on labeled data to train their algorithms. They need consistent, high-quality labels to ensure their models perform accurately and reliably in real-world applications.
- Healthcare Organizations: Medical research institutions and healthcare providers often use labeled medical images, such as X-rays or MRI scans, to train diagnostic AI systems. Accurate labeling in these applications is critical for identifying conditions and supporting medical decisions, ultimately improving patient care.
- Financial Services: Financial institutions use labeled transaction data, customer behavior insights, and sentiment analysis to power fraud detection systems, algorithmic trading models, and customer service AI. Precise labeling helps ensure these models are both accurate and compliant with regulatory standards.
- Retail and E-commerce: Companies in this sector use labeled images, text, and video data to enhance product recommendations, manage inventory, and improve customer experiences through visual search and product categorization.
- Agriculture and Environmental Science: Researchers in these fields require labeled satellite imagery, crop data, and environmental signals to monitor changes in ecosystems, optimize crop yields, and assess climate impacts.
- Autonomous Vehicles: Self-driving car manufacturers require vast amounts of labeled data, including images, LiDAR data, and GPS information, to train vehicle perception systems. Labeled data helps autonomous vehicles accurately detect and respond to obstacles, traffic signals, and pedestrians.
- Educational Institutions: Universities and research labs also require labeled datasets for developing AI and machine learning projects across a wide range of disciplines, from language processing to computer vision.
Data Labeling Pipeline
In the AI/ML pipeline, data labeling is a crucial step in preparing data for model training.
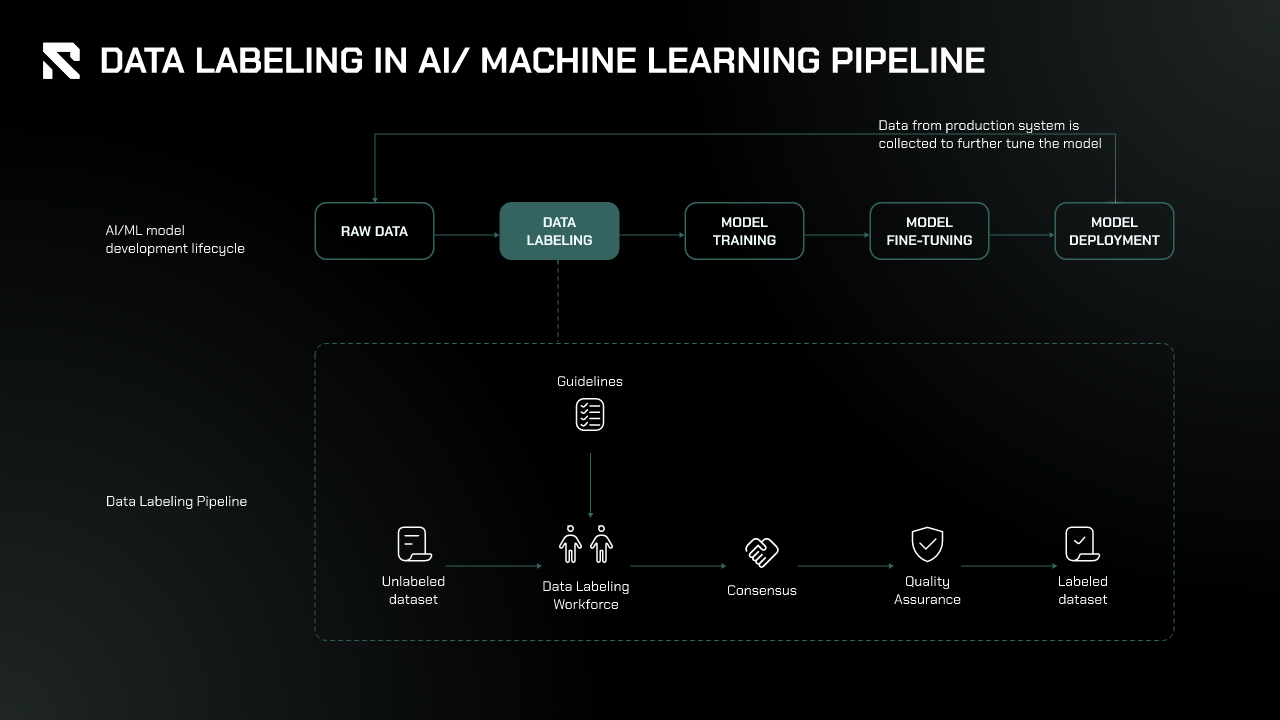
- Data Collection and Preprocessing: Raw data is gathered from diverse sources such as sensors, databases, and APIs. Since this data often lacks structure or format and may contain inconsistencies, it undergoes preprocessing, where it’s cleaned, formatted, and transformed to ensure consistency and compatibility for labeling.
- Data Labeling: After preprocessing, the data is labeled or annotated to provide the AI/ML model with the necessary information for learning. Labeling techniques vary depending on the data type, such as image, text, audio, or video. After the data is labeled, the Consensus and Quality Assurance (QA) stage ensures the accuracy, consistency, and completeness of the labels.
- Model Training and Fine-Tuning: The labeled data is then used to train the model, where it learns patterns between inputs and labels. The model is tested with unseen labeled data, and its performance is measured through metrics like accuracy, precision, and recall. If the model’s performance is suboptimal, adjustments are made before retraining, which may involve improving the quality of the labeled data.
- Deployment: Finally, the model is deployed into production, ready to interact with real-world data and generate insights or predictions.
Data labeling is essential in this pipeline, enabling models to learn effectively from high-quality, consistent, and structured data.
How Rynus fits:
- Data Labeling Workforce: Rynus can scale up to millions of Workers participating in data labeling tasks on the Telegram Mini App via any device (mobile, tablet, PC, laptop). This offers unprecedented scalability for data labeling projects.
- Labeling Quality: Rynus implements a credit scoring mechanism using error-trap data and the Rynus Consensus Zero Knowledge Proof. This approach helps verify and maintain high labeling accuracy by assessing and improving Worker reliability over time.
- Data Security: To protect sensitive data, Rynus employs a cut-and-mix data mechanism that allows Requesters to choose their preferred security level when uploading data.