In the AI/ML field, AI models were once the central element, but today, data has become the most critical asset – Data is the New Oil. Yet, data alone isn’t enough; standardized data (labeled data) has become the true bottleneck in AI training. Properly labeled data can boost AI training efficiency by 5-8 times or even more.
Therefore, having access to more high-quality data offers a significant advantage in the AI race. It’s estimated that up to 80-90% of an AI project’s time is spent on data-related tasks, such as labeling and annotation, with only 10-20% left for actual model training.
The success of any AI or machine learning model depends not only on advanced algorithms but also on the quality of the data it’s trained on. For these models to make accurate and reliable predictions, they need vast amounts of well-organized, correctly labeled data. Without this, even the most sophisticated AI can produce inaccurate or biased results.
This is where Data Labeling becomes essential. Properly labeled data allows AI models to understand and interpret real-world information, transforming raw data into a format that AI can learn from. Inaccurately labeled data, on the other hand, can severely hamper model performance, leading to costly errors and inefficiencies.
However, the process of data labeling – especially at scale – can be challenging. While automated tools can help, they often fall short in terms of nuance and context, missing important details that only a human can recognize. This creates a gap that needs to be filled with human intelligence to ensure that AI models are trained on the best possible data.
Three current major challenges in the Data Labeling and Annotation industry are:
- Quantity of Workers: Traditional data labeling companies can only engage a maximum of around 10,000 workers per project, limiting the scalability and speed required for large-scale AI training.
- Quality of Workers: Worker quality is typically controlled through human oversight, which does not guarantee consistent quality and is prone to human error.
- Data Security: Customers are often required to provide all their data to labeling companies, exposing sensitive information to potential leaks and compromising data security.
To address this need, Rynus offers a vital service: Rynus Data Labeling. By leveraging the power of human intelligence and innovative technology, Rynus ensures that raw data is accurately labeled and primed for AI/ML model training, delivering the quality needed for success.
Rynus tackles the three primary challenges in data labeling and annotation – Quantity, Quality, and Data Security – with the following solutions:
- Quantity: Rynus can scale up to millions of Workers through the tap-to-earn/listen-to-earn feature on its Telegram Mini App, offering unprecedented scalability for data labeling projects.
- Quality: Rynus implements a credit scoring mechanism using error-trap data and the Rynus Consensus Zero Knowledge Proof algorithm. This approach helps verify and maintain high labeling accuracy by assessing and improving Worker reliability over time.
- Data Security: To protect sensitive data, Rynus employs a cut-and-mix data mechanism that allows Requesters to choose their preferred security level when uploading data. For example, they can split images into 9 – 12 parts or smaller and choose to mix these fragments, ensuring no single Worker has access to the complete image and minimizing the risk of information leakage.
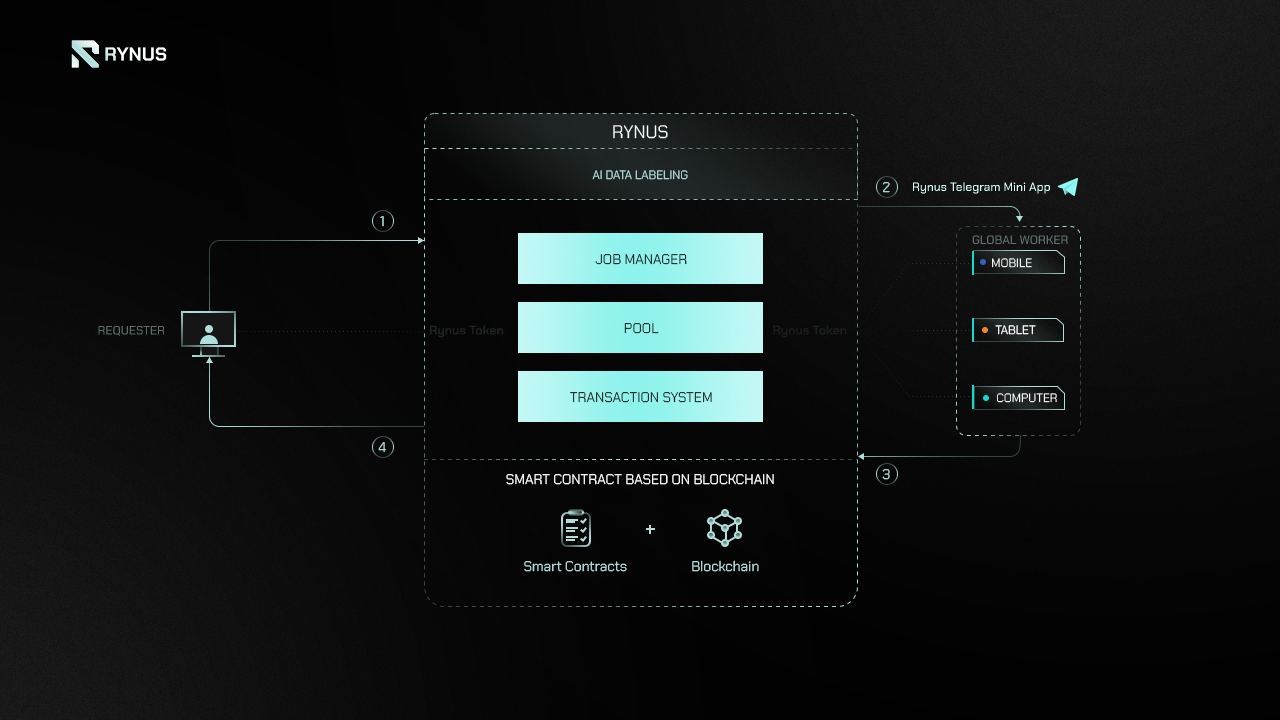
What is Rynus Data Labeling?
Rynus Data Labeling is a crucial service offered by the Rynus Network. It is designed to provide high-quality data annotation for AI and machine learning projects. In addition to its powerful computing services for AI training, Rynus stands out by offering a human-driven data labeling solution that ensures precision and reliability.
Unlike many platforms that rely heavily on AI for labeling tasks, Rynus taps into its decentralized human workforce, known as Labeling Workers (any device: mobile, tablet, PC, laptop), to manually label datasets. This approach addresses the common limitations of AI-based labeling tools, which can misinterpret complex or nuanced data. Human labelers bring a deeper understanding of context, subtlety, and detail, ensuring that the labeled data is accurate and ready for AI model training.
By leveraging this combination of human intelligence and decentralized infrastructure, Rynus ensures high-quality data labeling at scale, supporting clients who need large datasets labeled precisely and efficiently.
Who Uses Rynus Data Labeling?
Rynus Data Labeling is tailored for any organization or individual working on AI and machine learning projects that require high-quality, meticulously labeled data.
Key Features and Functionalities
Rynus Data Labeling offers several critical features designed to meet the data annotation needs of AI/ML clients across different industries:
1. Human-Powered Labeling for Precision
We rely on our decentralized network of Labeling Workers (any device: mobile, tablet, PC, laptop) to manually label datasets. This human-led approach ensures accuracy and avoids the errors common with AI-based auto-labeling solutions, especially when dealing with complex or ambiguous data.
2. Multiple Labeling Services: Image/Text/Audio/Video
- Image Labeling: Workers accurately annotate images, ensuring that objects, regions, or features within the dataset are correctly identified. This service is particularly useful for industries such as autonomous vehicles, security systems, and healthcare, where object detection and segmentation are critical.
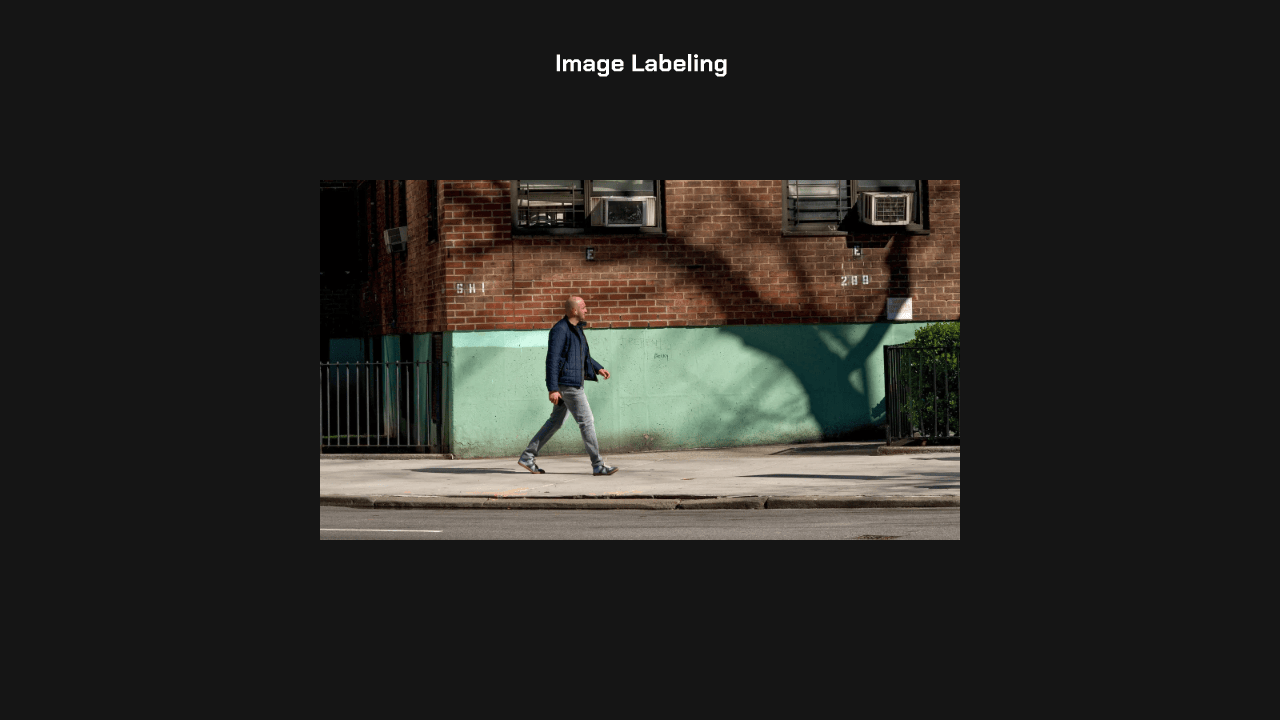
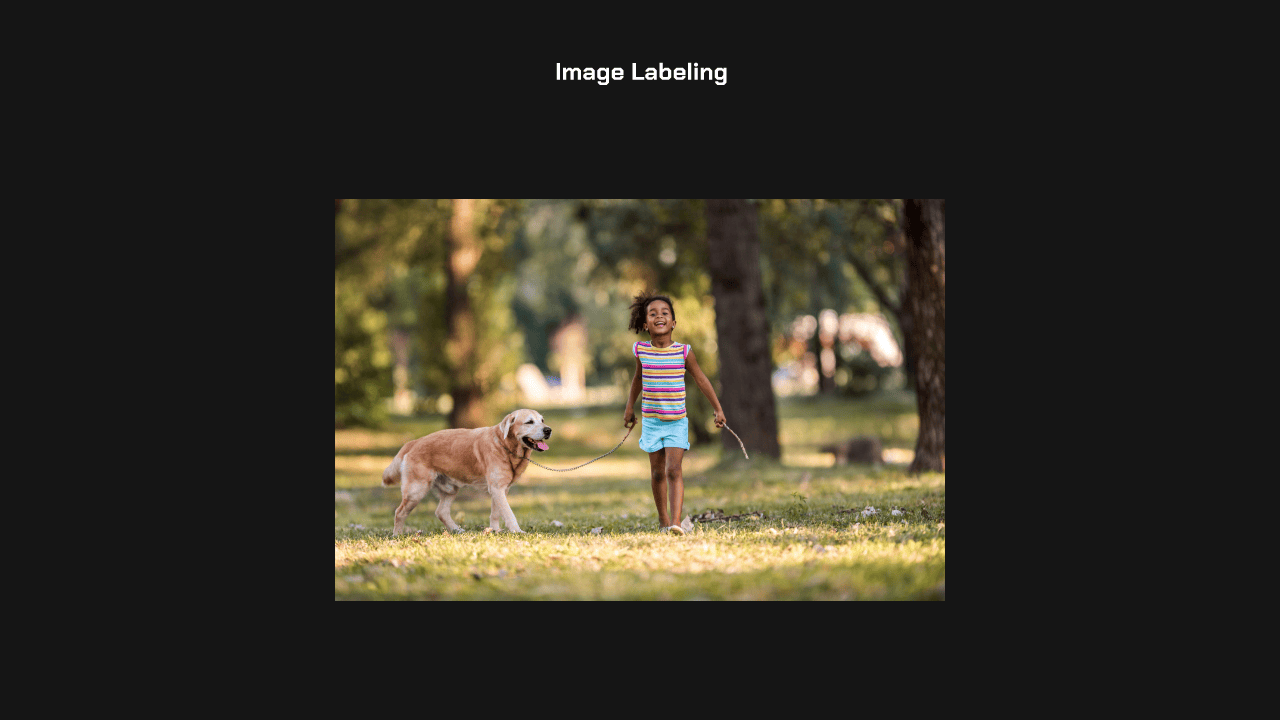
- Text Labeling: Workers label textual data, such as categorizing content, tagging named entities, and annotating sentiment. Text labeling is critical for applications like natural language processing (NLP), sentiment analysis, and AI-driven customer service, where understanding context and language nuances is key. This service is vital for chatbots, automated sales, customer support, translation, education, consulting, and more.
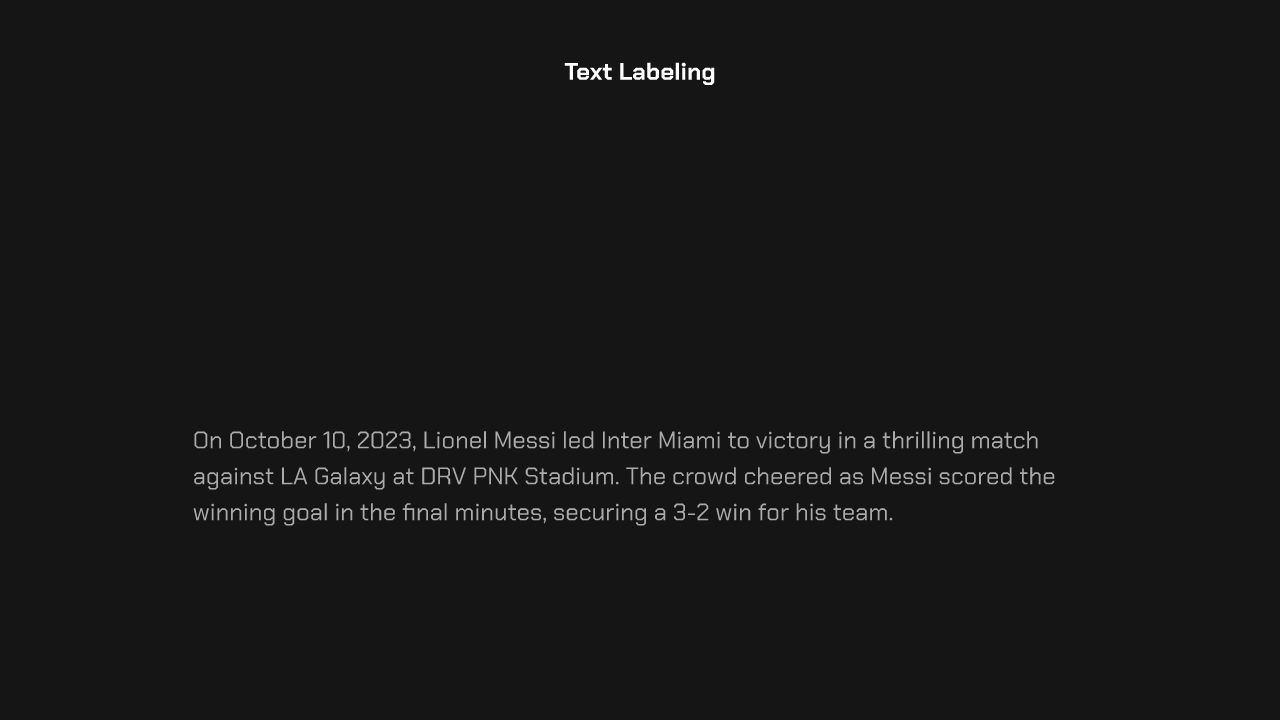
- Audio Labeling: In this service, Workers annotate audio files, tag spoken words, identify different speakers, and recognize background sounds. This audio labeling service is essential for applications like speech recognition systems, virtual assistants, and customer service bots.
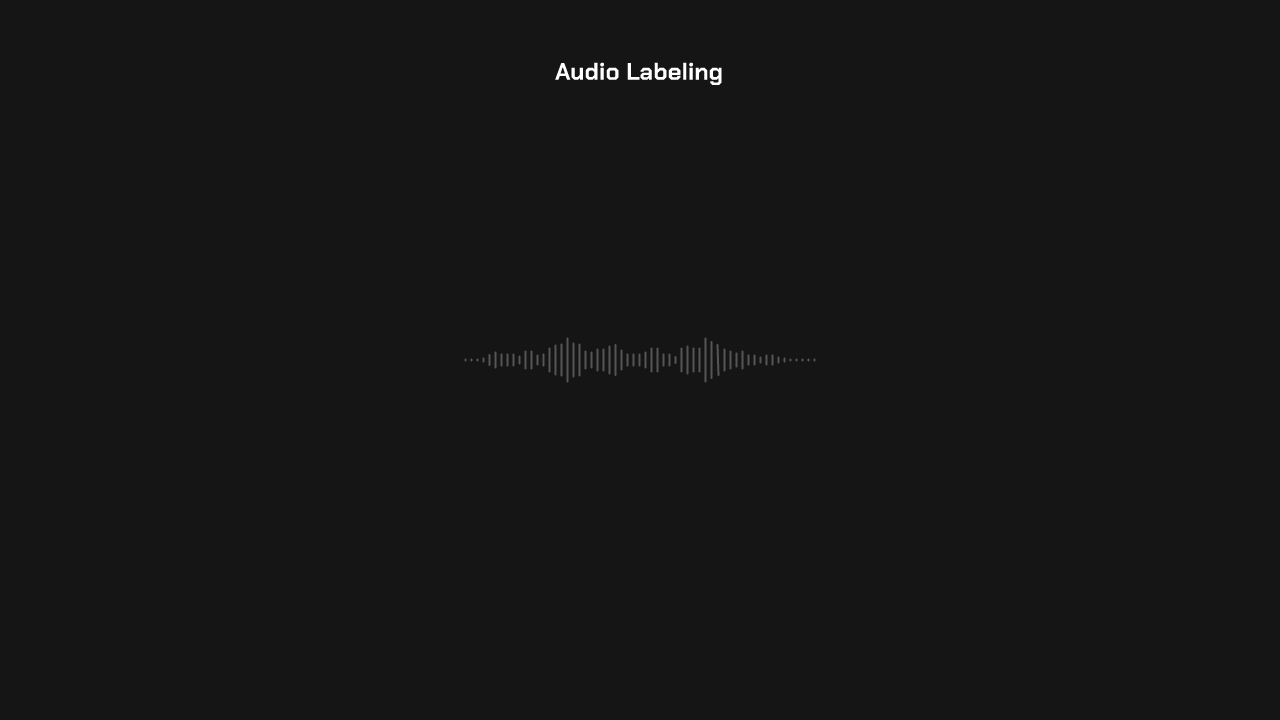
- Video Labeling: Workers annotate video footage, identify objects, track movement, and label scenes or actions within video datasets. This service is widely used in applications like video surveillance, sports analytics, and media content categorization, where motion tracking and action recognition are necessary.
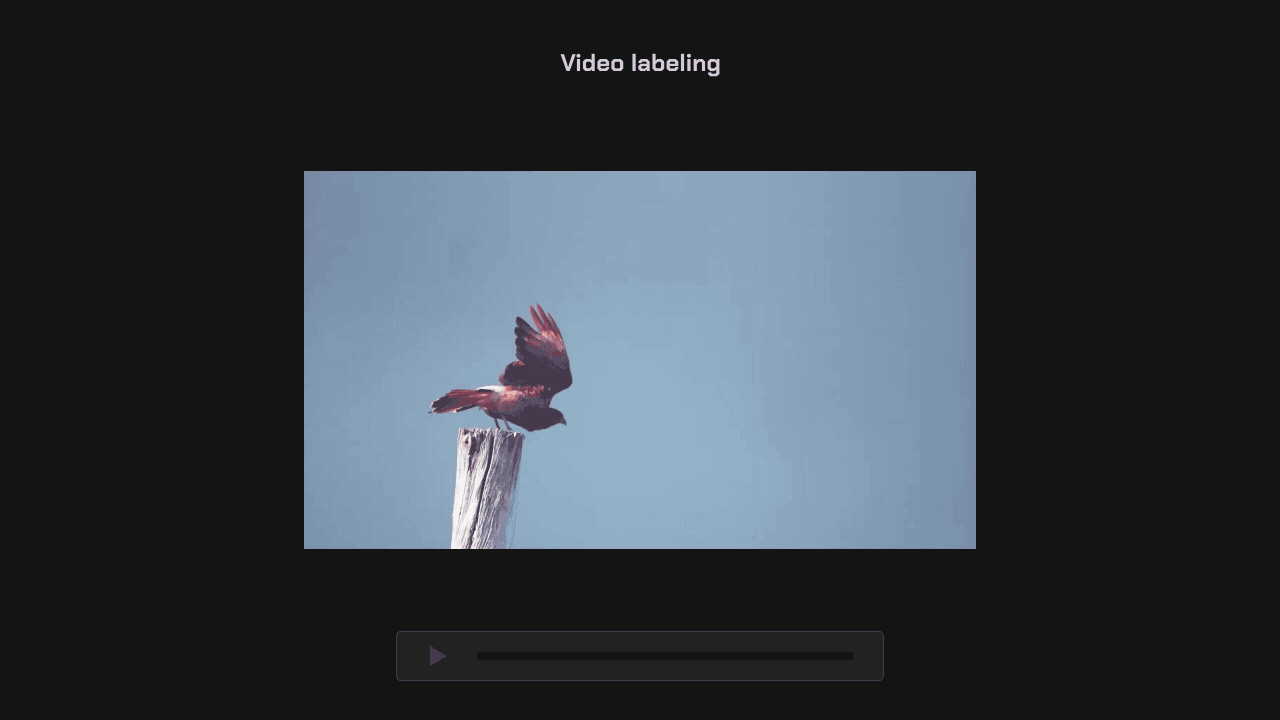
3. Decentralized Network for Scalability
We leverage our decentralized infrastructure to distribute labeling tasks across a global network of Labeling Workers. This decentralized approach enables Rynus to scale efficiently, processing large datasets quickly while maintaining high accuracy.
4. Credibility, Accuracy & Privacy
To ensure the highest standards of quality and security, Rynus employs advanced technology for the Quality Control and Review Process. Here’s how Rynus achieves this:
- Data Division and Mixing: To protect data privacy, Rynus divides and mixes data (for example, images) before they’re sent for labeling. This way, no individual Worker sees the complete picture, enhancing data security while still allowing efficient processing.
- Error-Trap System: Rynus integrates a unique error-trap system to maintain high-quality community contributions. When labeling tasks are distributed, 50% of the data initially contains “trap data” to assess and adjust each annotator’s credit score based on their performance. As annotators’ credit scores rise or fall, the trap data ratio will be adjusted to ensure continual accuracy.
- Consensus Proof (based on the Zero Knowledge Proof Concepts): This technology enables secure data processing without disclosing details. Rynus can accurately determine the correct answer from 51% of consensus annotators with high credit scores. When an image is labeled, it will be sent to a certain number of annotators. If the result matches among more than 51% of those individuals, it is considered the correct result.
5. Customizable Labeling Services
We offer flexibility in our labeling services to meet the varied needs of our clients. Whether clients need image classification, object detection, segmentation, or audio classification.
In summary, Rynus Data Labeling is an indispensable service for clients who require reliable, high-quality data annotations for their AI/ML projects. By combining human intelligence with a scalable, decentralized platform, Rynus delivers accurate data labeling that meets the demands of modern AI applications.
For Labeling Requesters, refer to Get Started with Data Labeling guide.
For Labeling Workers, refer to Get Started Mini App guide.